On the way to efficient phenotyping for Germany’s next wheat variety…
Sustainable crop production requires knowledge of the structural and functional status of plants in the field in order to plan and implement precise measures. Although significant progress has been made in sensor technology, machine learning and autonomous robotics in recent years, we are still at the beginning.
In Use Case 5 “Non-invasive phenotyping with autonomous robots”, FAIRagro is investigating the potential of multimodal data analysis methods and machine learning algorithms for phenotyping plants in the field. You can find out about the current status of Use Case 5 and what the future holds in this report.
Contents of this page:
In this Use Case Update, you can find out what role fair research data management plays in this, what steps the Use Case 5 team has already mastered and what challenges still lie ahead. We show why sensor systems play a major role in phenotyping – and what phenotyping actually is. You can also find out what FAIRagro’s collaboration with scientists in these areas looks like in concrete terms.
In Part 3 you will find valuable hints about potential connections for future Use Cases – probably yours?
UC 5 Partners


Motivation
Finding Germany´s next wheat variety
In today’s agriculture, it is just as important as in plant breeding to quickly and efficiently record how plants “perform” under different environmental and management conditions. A key tool for this is phenotyping – the characterisation of plants based on specific traits.
Traditional methods vs. modern technologies
While plants traditionally had to be painstakingly “harvested” by hand and broken down into their individual parts to determine certain characteristics, such as plant height and biomass, the use of sensors and the automation of processes now allows much higher throughput rates and therefore much broader application possibilities.
Progress through sensors and automation
With the introduction of non-invasive phenotyping technologies, combined with robotics and remote sensing techniques, scientists and practitioners can now work more efficiently. These technologies, which are being developed and refined by the Use Case 5 team, facilitate informed and evidence-based decisions.
Advantages for breeders and farmers
These advanced methods not only support breeders in their search for the best wheat variety for cultivation in Germany, but also help farmers in their daily management decisions in the face of changing conditions and climate change.
Phenotyping and sensors – It´s a match
Remote sensing, different sensor systems and automation play a central role in modern phenotyping. These technologies make it possible to measure important characteristics and calculate valuable indicators in real time – from the leaf and plant level to the field or even landscape level.
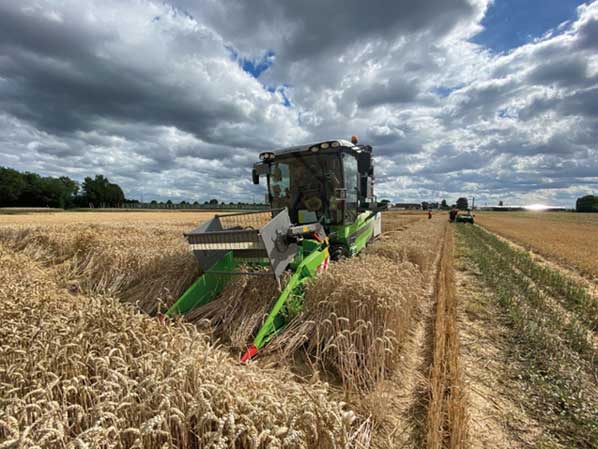
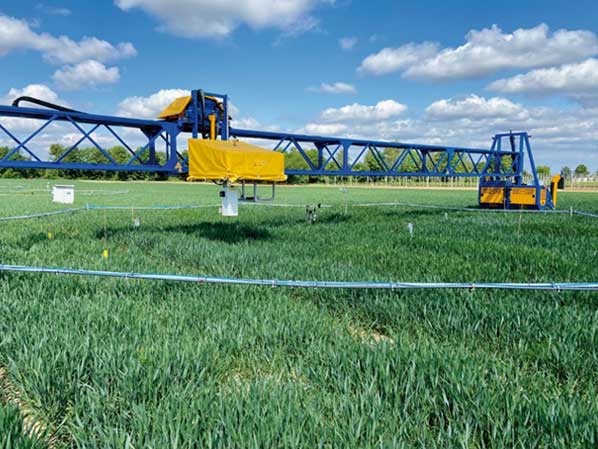
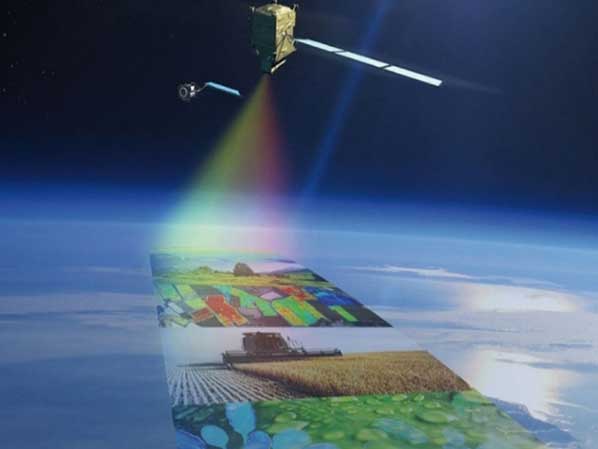
Different phenotyping platforms in practical application.
Source: Measuring, scaling, and understanding solar-induced fluorescence from leaf to the canopy and field scale, Forschungszentrum Jülich
Chlorophyll fluorescence as an indicator of photosynthetic performance is an illustrative example of sensor measurements and indicators of plant performance derived from them. An overview and latest findings on “Measuring, scaling and understanding sun-induced fluorescence from leaf to canopy to field scale” was presented by Uwe Rascher (Forschungszentrum Jülich (FZJ), head of UC 5, in a presentation at the Flex Fluorescence Workshop in 2023.
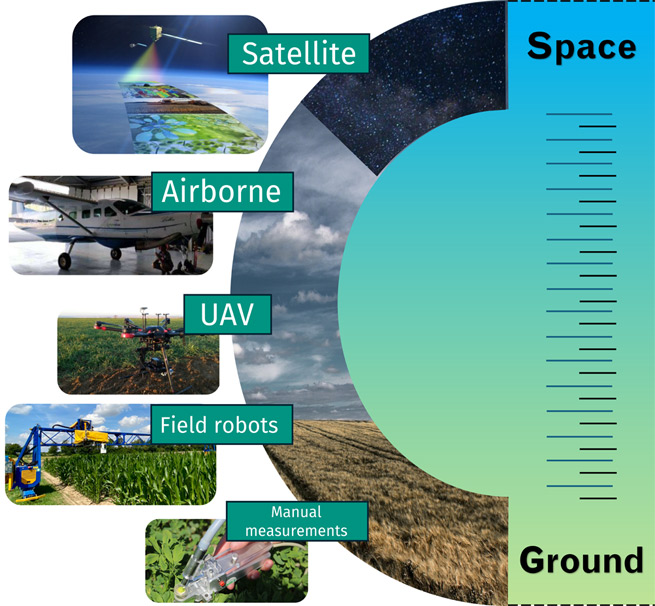
Sensor systems with instruments for the quantification of fluorescence for non-destructive field phenotyping on different scales. Sources: European space agency, Forschungszentrum Jülich, Pixabay (Modified).
Autonomous sensor measurements in agriculture
Nowadays, sensor measurements no longer have to be actively carried out by humans on the plants. Instead, they can be carried out autonomously in and above the field. Sensors are attached to agricultural machinery that drives across the field or installed on drones and aeroplanes that fly over the landscape.
Innovation through satellite technology
A significant advance in remote sensing will take place in 2026 when an ESA satellite is launched. Equipped with the “FLORIS” imaging spectrometer, this satellite will enable global fluorescence measurements and thus provide even more comprehensive data on plant performance.
The calibration and validation of sensors requires data – lots of data 🙂
The calibration of new instruments and sensors as well as the validation of measurements require access to high-quality data sets. The publication of such data sets is of great importance. By combining different data sources and types, scientists can now determine complex indicators with increasing accuracy. This significantly improves the reliability of predictions of plant performance under changing conditions.
Achievements of UC 5
Use Case 5 (UC 5) is committed to creating essential prerequisites and specifying the requirements for research data management (RDM) for the publication of comprehensive phenotyping datasets. As an important step, a set of several benchmark datasets with field phenotyping data has now been published. This will form the basis for further research and the development of more precise phenotyping methods.
Preliminary achievements
The Repo Reality Check
Improving data availability through publication in data catalogues
A crucial step in improving the discoverability and accessibility of datasets is their publication in non-proprietary data catalogues or repositories. The UC 5 team conducted tests on various subject-specific repositories to assess the requirements for publishing multi-sensor datasets.
Challenges in the publication of heterogeneous data sets
Although numerous subject-specific repositories exist for the publication of data in agricultural systems research, the effectiveness of publishing heterogeneous datasets (such as images, phenotypic IDs, sensor IDs, aerial photographs, soil data, etc.) remains challenging in practice. Are the available datasets fully describable with metadata? Do the existing repositories fulfil the FAIR criteria for data publication?
Evaluation of repositories and derivation of recommendations
The UC 5 team intensively tested various repositories, including OpenAgrar, Bonares, PhenoRoam and Jülich DATA, to find out to what extent heterogeneous multi-sensor data can be published in accordance with the FAIR criteria. For example, it was examined which legal requirements must be met so that participating scientists can publish comprehensive data, which licences can be used or selected and which possibilities or restrictions this entails for subsequent use. Particular attention was paid to the metadata and it was critically scrutinised whether this sufficiently depicts and describes the data so that subsequent use and simple “data harvesting” become a reality. Recommendations for remote sensing-specific metadata were derived from this, which are to be taken into account in the metadata and standardisation developments of FAIRagro.
Milestone: Publication of several comprehensive Bench mark data sets
Following the publication of a first benchmark dataset by the UC5 team in 2023, the publication of a set of several comprehensive benchmark datasets in December 2024 marks a significant milestone.
The benchmark data set of December 2023: Field Phenotyping of Ten Wheat Cultivars
Adaptation of RDM infrastructures to the needs of researchers
The first version of the benchmark dataset has already been presented in a DeepDive with the NFDI consortium DataPLANT and used to test the annotated research context (ARC) tool ARCitect. As a central component of the research data infrastructure that is currently being developed, ARCitect should later enable the “harvesting” of data from various repositories, data catalogues and banks.
The intensive check of the repositories was an important step in adapting the RDM infrastructures to the needs of researchers and meeting the objectives of FAIRagro. The aim is to further develop services and infrastructures for the community.
Collaboration with data stewards for support and training
Collaboration with the Data Stewards of the Data Steward Service Centre (DSSC) is an important building block for this. FAIRagro data stewards were not only able to support UC 5 with their expertise, but also help to pass this on to the community as part of joint training sessions.
Integration of UC 5-results in FAIRagro-Trainings
The UC5 team also played a key role in organising a FAIRagro training course led by the FAIRagro training coordinator on the topic of “Handling and organising plant phenotyping data – a workshop on Research Data Management” for interested parties, thereby disseminating the results of the FAIRagro UC work to the community.
Next steps & points of connection for new UC
Richy rich metadata (models)
The Repo Reality Check is a central preliminary work and provides important experience for the annotation of research data as well as for the demand-orientated further development of extensive, i.e. “rich”, metadata.
Importance for FAIR data concepts in agricultural systems research
On the one hand, this is important for the work of Task Area 3 (TA), which aims to consolidate FAIRE data concepts on the basis of metadata models for scalable research data infrastructures and thus develop guidelines for FAIRE data publication in agricultural systems research.
Integration of scientific workflowsI
This in turn is an essential prerequisite for the integration of scientific workflows that enable automated data curation or simplify data visualisation, among other things. Future UC can also help to sharpen the needs of the community and support the implementation and testing of specific workflows in order to make innovative research data management a tangible experience for researchers.
Potential for Precision Farming und Machine Learning
There are interesting points of contact, particularly in the fields of remote sensing and computer science. How can the potential of better data availability be utilised in precision farming and what role do machine learning methods play in this? These questions open up exciting application possibilities for practice-orientated agricultural research. Automated analyses of large and high-quality data volumes can enable more precise management decisions or accelerate the selection of new wheat varieties adapted to climate change.
by Irek Kleppert and Anne Sennhenn, April 2024