Revolutionizing Crop Model Applications through FAIR Research Data Management
Discover how FAIR research data management is transforming crop model applications: Read about the steps the Use Case 6 team has taken, the challenges they face, and the potential for further advancements. This report begins with an overview of the limitations and applications of crop models.
It also highlights the collaboration between FAIRagro and scientists in this field. Finally, it outlines the next steps towards innovative crop model applications. Make sure to read to the end and consider how FAIR research data management can revolutionize your daily life as a researcher.
Contents of this page:
Feel free to share your own data management challenges and join FAIRagro with your own use case!
UC 6 Partners

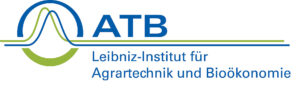

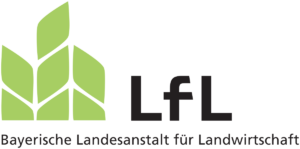
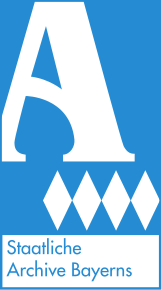
Motivation
Crop Models dress up for future applications
Crop models serve as a toolbox for agricultural researchers, using equations to simulate or predict how crops will respond to various factors, ranging from environmental conditions to management practices and crop or variety selection. Traditionally, these models have been employed to forecast crop performance under different environmental circumstances.
The effectiveness of these models—whether for calibration, validation, or application—depends on high-quality input data. This includes weather parameters such as temperature, rainfall, and solar radiation; soil characteristics like texture and water content; farming inputs such as planting dates and fertilization; crop growth observations and measurements like flowering date and yield; and even plant genetic traits like sensitivity to vernalization or photoperiod. The necessary data are highly diverse, and their collection—including data search, formatting, and quality control—requires significant time and resources. Although these models could theoretically support decision-making in crop management, such as by modeling changes incrop growth in response to upcoming weather, practical application is rarely feasible due to the cumbersome nature of data processing.
“Harvesting” data from various resources, including domain-specific repositories and generic databases like the DWD (Deutsche Wetterdienst) Open Data Server, is a prerequisite for establishing automated data acquisition and processing workflows. However, despite the seemingly easy and fun connotation of “harvesting” data, the reality is quite different. There are several hurdles for convenient data acquisition, including:
- Scattered and diverse data sources, limited data findability, and accessibility
- Lack of standardized vocabularies for data description
- Inadequate or missing data documentation and metadata standards
- Limited knowledge of research data management among crop modelers
The Use Case 6 team aims to take a step further by enabling and combining the application of existing data with real-time data from sensors in the field and implementing scientific workflows that integrate different data sources, allowing for advanced model applications.
Use Case 6 makes it possible to grasp and experience the practical possibilities that FAIR research data can open up for scientists and users.
We are more than excited about it ☺
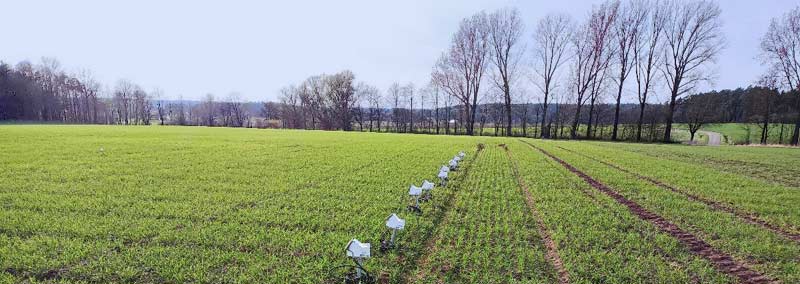
Preliminary achievements
Data Harvesting Action Plan
Establishing Minimum (Meta)Data Requirements
The first step towards maximizing the potential of crop models is defining the essential (meta)data requirements for crop modeling and advanced applications. Key metadata attributes—such as cultivar, maturity date, and yield—were identified to facilitate the retrieval of crop experiment datasets containing the necessary data for modeling applications. The goal is to provide data infrastructures with relevant data properties to improve the quality of search results for crop modelers.
Harmonizing Metadata Standards
The UC6 team has identified established FAIR (meta)data standards and vocabularies to harmonize the annotation of crop experiment data. For the publication and archiving of crop experiment data, structured vocabularies such as AGROVOC and the BonaRes LTE data model, covering both English and German languages, were identified as effective human-readable and well-established standards in agriculture. By promoting the use of these structured data vocabularies, UC6 aims to standardize the published crop experiment data, facilitating its integration into data processing pipelines and enhancing its potential for reuse. The UC6 team is also engaged with FAIRagro and the DataPlant consortium, providing example datasets to design FAIR digital objects documenting the entire data lifecycle.
Designing Standard Workflows for Data Integration
Crop models require extensive data. To facilitate the reuse of third-party data, Use Case 6 focuses on designing standard workflows to extract, transform, and integrate data from repositories specialized in crop experiments (e.g., BonaRes repository), weather (e.g., historical and forecast data from the DWD Open Data Server), and soil profiles (e.g., National Agricultural Soil Inventory) into input formats for modeling frameworks.
For modeling applications, the ICASA standard, widely accepted among the crop modeling community, serves as the ideal intermediate step for data transformation. It can be readily translated into input formats for multiple crop modeling frameworks, such as DSSAT or APSIM. This translation process, also called data mapping, involves converting data from various sources into a specific structured format, including name matching, unit conversion, and variable derivation. The UC6 team collaborates with the Agricultural Model Intercomparison and Improvement Project (AgMIP) initiative, which has developed tools to facilitate data mapping into the ICASA format and subsequent translation into input formats for several established crop models. As of 2024, a full data integration and simulation pipeline based on prototype datasets has been developed. Current work aims to generalize this approach to all datasets annotated following the BonaRes LTE data model.
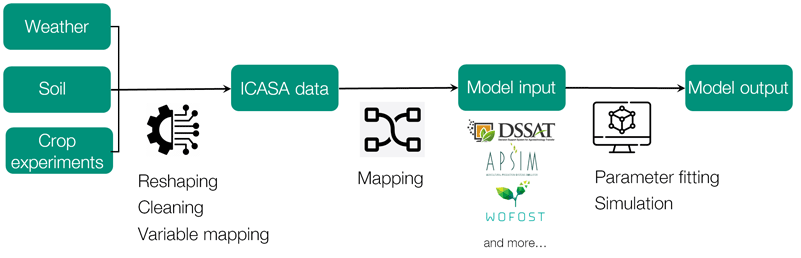
Improving Data Quality Control
In addition to data integration and formatting, the UC6 team is developing tools to enhance data quality control in crop modeling applications. These tools will include implementing a published scoring system to assess the fitness of crop experiment data for use in the calibration and validation of crop models. Interactions with crop modelers help inventory routine quality control and error correction procedures and their associated data quality challenges. This work will be shared with data quality experts in the broader consortium to support the development of quality control tools within the FAIRagro infrastructure. Furthermore, the team is developing a prototype workflow for fitting genetic parameters in the Nwheat crop model, which constrains estimation algorithms with expert knowledge to ensure the use of biologically accurate genetic traits in crop models.
Ensuring International Interoperability
Thereby UC6 not only provides practical requirements for data quality necessary for the development of useful FAIRagro services but also ensures connections to international initiatives, promoting interoperability on an international scale within the research community and beyond.
Streaming data from the field to simulation models
UC6 focuses on developing rapid data streams “from field to simulation” to showcase the potential of crop models as decision support systems in crop management. In 2024, the UC6 team initiated a pilot field trial where crop growth, soil, and weather data are collected via various sensor technologies, including soil probes, weather stations, and UAVs. These input data streams are connected to the previously described workflows through a dedicated web application.
The goal is to automatically integrate field data with experimental metadata (e.g. management inputs) and third-party data (e.g., weather forecasts) to perform crop growth simulations with the DSSAT crop modeling framework. This experiment is designed as a foundation for establishing robust data streams that will be scaled up in a large-scale field trial in 2025. The aim is to test the potential of predictive crop growth simulation to support short-term decision-making in crop management.
Comprehensive metadata annotation
The Smart Rural Area Data Infrastructure (SRADI) is a research data management infrastructure dedicated to documenting metadata associated with agricultural research project at the Technical University of Munich and partner research institutions, based on recognized international standards such as OGC, ISO or DCAT. SRADI comprises the aforementioned sensor management we application (Sensorhub) and a metadata catalogue (Agrihub). Digital twins of all resources involved in the project (for example, field plots, sensors, web services, workflows, datasets…) are gradually registered into a data catalogue (SRADI Agrihub) to allow a comprehensive and transparent annotation of the data generated throughout the project. This is a crucial step to ensure that all data produced during the experiment are richly documented and fit for publication and long-term archiving in compliance with the FAIR principles.
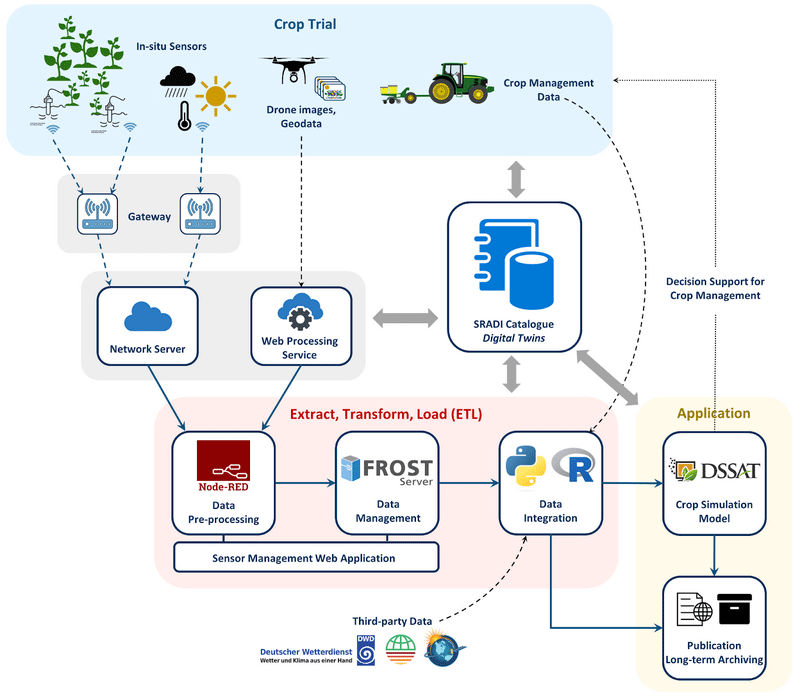
Next steps & points of connection for new UC
Crop models on the catwalk
Next Steps in Data Management for Crop Models
The next steps include establishing a long-term archiving pipeline to foster the findability and reusability of the wealth of data produced by agronomists at the Bayerische Landesanstalt für Landwirtschaft (LfL). . These data have great potential for reuse in crop model application, though this potential is presently wasted by the lack of systematic documentation and archiving processes. To make data from LfL available for research, we organize a data management workshop, scheduled for autumn 2024, under the lead of the Bavarian State Archives, in partnership with education and training experts of the FAIRagro consortium. This workshop aims to extend concepts to make agricultural data from agricultural trials findable, accessible and reusable for agrosystem scientists in accordance with data governance policies. Additionally, it will focus on establishing a concept to systematically archive field trial experiments. Implementation of metadata standards are crucial and will be a key focus of the planned workshop.
FAIRagro Joint Venture with SRADI
Another FAIRagro joint venture involves connecting the Smart Rural Areas Data Infrastructure (SRADI) of the World Agricultural Systems Center/Hans Eisenmann-Forum für Agrarwissenschaften (HEF) at TUM to the FAIRagro search portal. This collaboration will enrich the existing FAIRagro while enhancing the findability of agrosystem research projects conducted by Bavarian research institutions. We are excited to implement innovative scientific workflows and make them accessible to a broad community. This approach will enable cutting-edge model applications with great potential for researchers and practitioners.
by Benjamin Leroy and Anne Sennhenn, May 2024